Bold action and novel approaches
There comes a time in the trajectory of any innovation when its use without meaningful governance becomes untenable. Consider automobiles, first seen as transformative and disturbing. Concerns about their safety fell after speed limits and traffic lights became law. In its early days, innovation flourishes when unconstrained by rules. A hands-off approach allows inventors to dream big, to create the previously unimaginable. But as the pace, scale and mix of applications from an innovation grow, so too do its expected and unexpected risks and ripple effects.
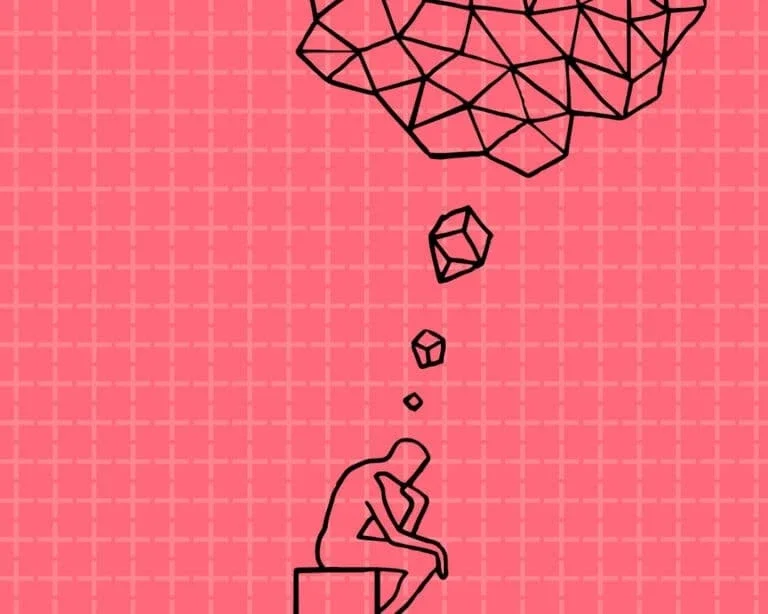
Wise, calibrated interventions at this inflection point – when concerns from the public begin to outweigh its need for unfettered innovation – are critical, particularly if an innovation has a range of both potentially positive and negative impacts, often unequally distributed. Such interventions, or governance, standardize the rules of the game for a level and predictable playing field on which many sorts of players can strategize, meet goals, and avoid penalties.
From helping find lost children through facial recognition to using the technology in satellites to identify Roman pottery shards, it is time to develop artificial intelligence (AI) governance in equally novel ways. Increasingly, bright minds in the AI field welcome such interventions to help correct for perverse power imbalances, to rein in AI-induced harm, and to expand markets for positive AI-driven goods and services. Effective AI governance will help us solve hard problems in our ecosystems, cities, health and other areas.
History shows us that governance applied at the right time, in the right manner, can help accelerate technical progress, creating business opportunities, and ensuring positive social outcomes. Pharmaceutical approval processes, for example, materially expanded work on medical cures because drug makers knew that independent agencies assumed the responsibility of ensuring the safety and efficacy of new medicines. Casualties of the uncontrolled use of AI show us the cost of inaction.
This year demonstrated AI’s myriad uses for good. AI helped free a despondent world from a pandemic through accelerated vaccine development and brought insurrection planners to justice via machine learning-generated evidence. At the same time, AI-driven misinformation campaigns, autonomous devices and racial profiling continue to alarm governments. The Rockefeller Foundation, as an institution deeply committed to equity, shares these concerns.
To help steer AI’s future towards benevolent purposes and to put AI’s many challenges on the global agenda, the Foundation in 2019 launched a series of convenings broadly focused on responsible AI. Experts from universities, think tanks, companies and nonprofits singled out AI governance – or the practices, pacts, processes and laws that track and react to the intended and unintended uses of AI and its follow-on impacts – as a vital first step towards shoring up its responsible use. As AI continues to course through every facet of our daily lives, the need for AI governance frameworks takes on increased urgency.
Appropriate and right-sized governance will allow AI’s use for good to grow through, for example, free and predictable markets, while reining in potential harms such as privacy intrusion or unjust outcomes from ingrained biases. Governance will also empower institutions and processes to halt AI’s use for malevolent ends. Striking the right balance between laissez-faire and targeted interventions isn’t easy. But we need this risk-reward tradeoff.
AI’s many applications and potentially profound outcomes, however, make its governance complex and use-specific. Governance therefore requires bold action and novel blended approaches that tap incentives, goodwill, expertise and efforts that are already underway. The Rockefeller Foundation seeks to be a catalyst for developing such AI governance models that help ensure that AI is used responsibly. We aim to forge critical connections between ideas and experts who can accelerate progress on AI governance efforts that might otherwise take decades. We are doing this through convenings, leadership support, funding for entrepreneurial collaboration projects, knowledge sharing, and network engagement.
What guardrails does the world require to rein in risks from AI? What solutions are possible? We hope to reframe AI as a set of technologies and tools with tremendous potential that demands governance to cross the chasm from broad general theory to clear, powerful action.
A nascent field of practitioners, several of whom we quote below, are exploring the common and disparate threads of ideas, networks, and practices to weave together their work-in-progress, and to push the frontier into flexible yet meaningful AI governance. Building on their momentum, in December 2020 we convened experts ranging from software safety auditors to lawyers, anthropologists, modelers, cyberpolicy specialists and policy intervention tool builders, to surface promising ways to address AI governance. Insights from those leading thinkers, below, shed light on realistic solutions and next steps to establish governance models.
Their ideas are presented in an iterative, open-source spirit, with suggestions for guardrails to direct the rollout of increasingly ubiquitous AI applications. Their questions help shape the contours of solutions that can harness AI for good while minimizing its harms:
- Do we need to bridge and plug existing networks? Or to create new oversight agencies like a Food and Drug Administration or Consumer Financial Protection Bureau to approve, monitor and impose penalties for AI use/misuse?
- Can we fight fire with fire through innovations like ‘Personal AIs’ – individual avatars that harness AI to query, prevent intrusion and demand recourse?
- Might we adapt consortium-led self-policing efforts akin to carbon footprint standards, such as ethical AI screens at the algorithmic design, project funding or approval stage – with crowdsourced shaming of violators?
- How can we integrate diverse perspectives on the need for and ways to address AI governance through tech-industry-ethicist ‘trilinguals’ on government and corporate technology teams?
- Can we conduct regular audits of AI software, algorithms or models?
- Would it be feasible to require government agencies to procure only goods and services with approved ‘safe’ AI? How might we establish those practices?
- What are the metaphors we should use to demonstrate AI’s awesome power and outcomes in simple, compelling ways — such as for autonomous weapons–to boost political and public understanding of AI and foment urgency for its governance?
- Do we need to develop an entirely new academic discipline at the nexus of computer and social science?
- Or do we need all of the above in an ‘AI innovation marketplace’, perhaps assigning governance to external specialists – in a parallel or staggered way?
Moving from lofty principles to actionable solutions on these and other important AI governance issues will take hard work. It is time for a community to test and launch new ideas and practices, to build a new AI governance field.
Build AI Governance models for real world, specific use cases.
Sweeping statements about AI, which is an opaque and complex set of technologies, block the progression of its governance from theory to use. Let’s get granular, exploring precise business use cases in search of clear, understandable governance models.
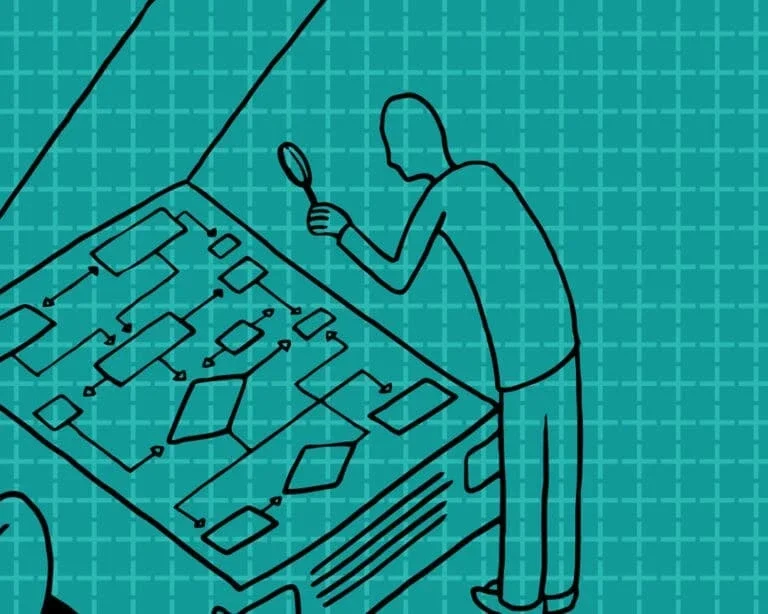
Solution Contours
Issues linked to the use of AI for, say, automated cars are quite different from those for jail bonds. Interdisciplinary teams with expertise in technology, industry, public policy, and ethics in different contexts digging deep into specific use cases will help accelerate the development and adoption of AI, writ-large, in many areas, building reference points for the field.
Adapt privacy and misuse laws and regulatory models to develop solutions we can use today
Building on existing laws that wrestle with similar issues of privacy and misuse may prove most effective at governing AI, at least short-term.
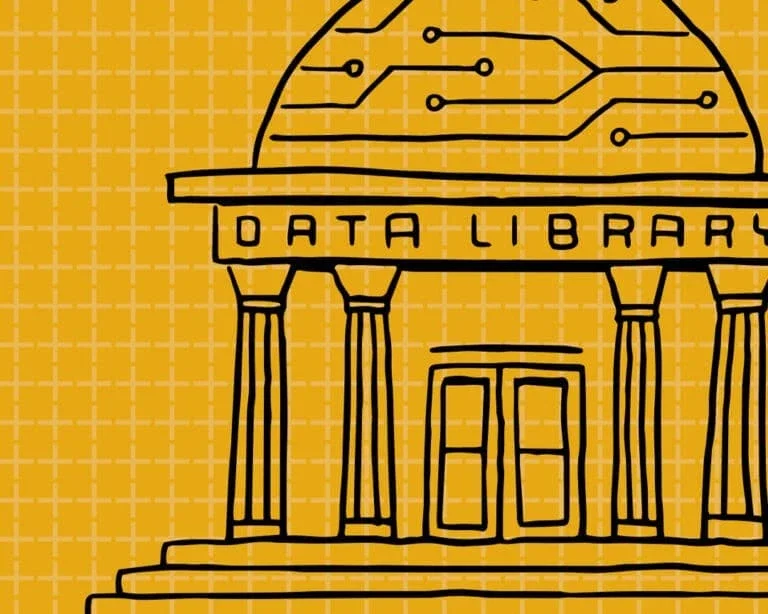
Solution Contours
Europe’s GDPR primarily addresses data privacy and security, not algorithms and models. It can serve as a base for AI governance with broader coverage over different areas including the right to reasonable inferences.
Reconcile the healthy tension between the need for global norms and local concerns.
Technology is increasingly developed quickly, with more complexity, in a globalized world. Platforms are global and their problems need to be solved in a global context. Yet most regulatory structures are at the national level and AI oversight needs to be culture specific. We should think big and broad, and about what is needed for global governance to work.
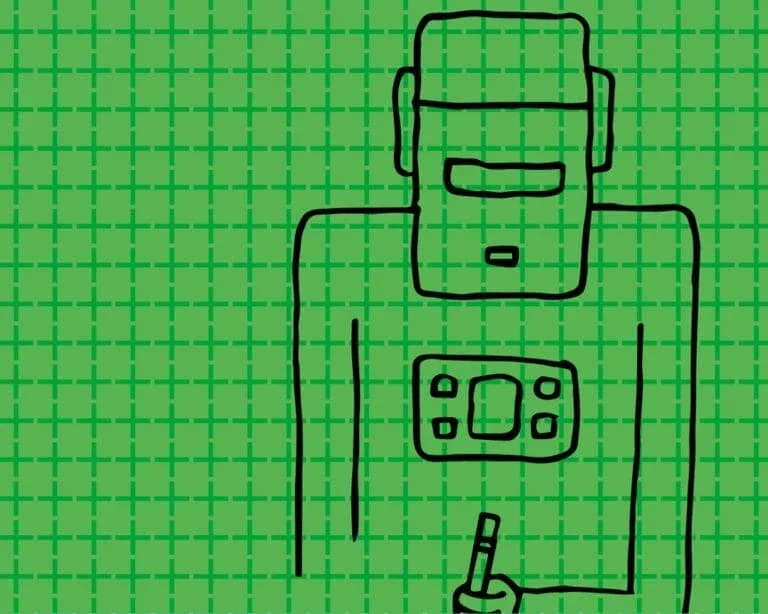
Solution Contours
Look to successful global regulations like those for bioengineering, Bretton Woods, the Forest Stewardship Council and Medical Device Single Applications. To what extent can we break them down into their attributes – including a public-private focus, non-state involvement, national security concerns, the possibility of opting in or out, and tradeoffs between sovereignty and hands-off – and use them as models for AI Governance?
Adapt pull vs push mechanisms from other sectors that have effectively minimized power imbalances.
When power rests in the hands of few, carrots can be far more effective than sticks. To encourage the positive and just use of AI, we need global incentives. Independent agencies that assess safety, efficacy, and fairness in certain products or services could serve as models for similar AI-focused agencies. They can provide guidance on how to address issues like harm or bias through an approval process or state intervention.
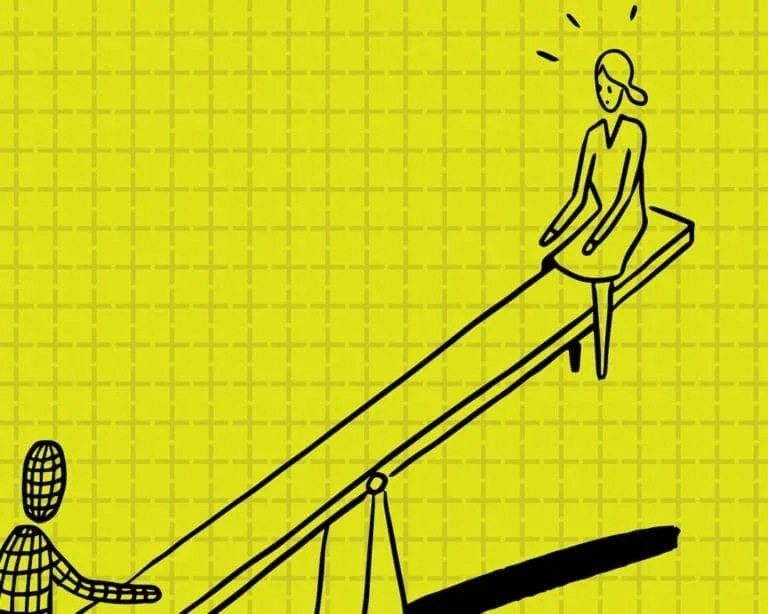
Solution Contours
Many proven domain-specific standards such as LEEDs certification, the Good Wood Scheme or Fair-Trade stamps motivate business leaders to do the right thing, with public watchdogs across the world holding them accountable. Still, questions remain: certify the company, the model, its level of safety or expected benefit? Institutions could define goals and metrics to address safety and possible adverse events, first testing AIs in priority sectors. This independent third-party could stipulate testable metrics in code through which a model must be run, for precise output metrics.
Ensure that all stakeholders, in industry, government, local communities and elsewhere speak the same language and have a shared understanding of AI’s capabilities and shortcomings.
Vast gaps in understanding AI between developers and governments make progress on AI governance extremely challenging. Connecting private companies producing AI tools with public sector bodies who plan to cooperatively regulate AI use in an open, honest, and shared language is the only way to ensure that AI governance reflects societal values.
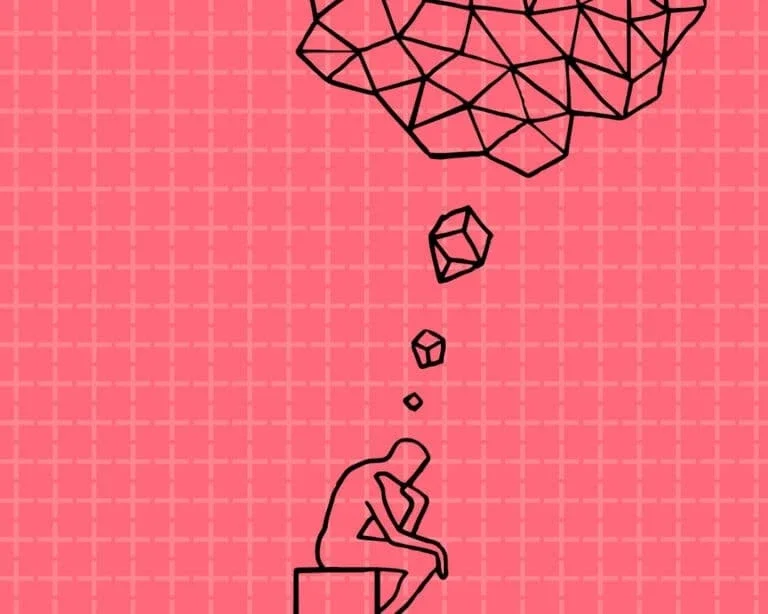
Solution Contours
Multi-sectoral coalitions of the willing to understand values, what’s at stake and how AI works. The consequences of misunderstanding in areas like weapons automatization or criminal law are too dire. Embedding technologists in government will help boost digital literacy and move AI governance from theory to practice. Technologists on public sector teams could also provide better information to the government and public on how AI transformations will impact or are impacting tangible lived experiences. ‘Bilinguals’ in Governance – those with domain and AI expertise, in areas such as health, could be very valuable. Conversely, interdisciplinary teams of social science researchers and tech developers at companies can push them to produce goods from both perspectives as teams grapple with questions like ‘What is fair AI between tech developers and regulators? What is a good explanation?’ Why not get tech teams to take on AI governance as a ‘Challenge’?
Integrate current thinking about the ethical and safe uses of AI to boost the efficacy of its governance.
Safety and ethics should be an embedded cost for development teams. Required reporting on the intended uses and possible repercussions of algorithms, models and data collection and use in the design phase of a good would help contain AI’s unfettered application as these products or services are developed. Historical antecedents in technology that have tested for harm in the early stages of development can serve as reference.
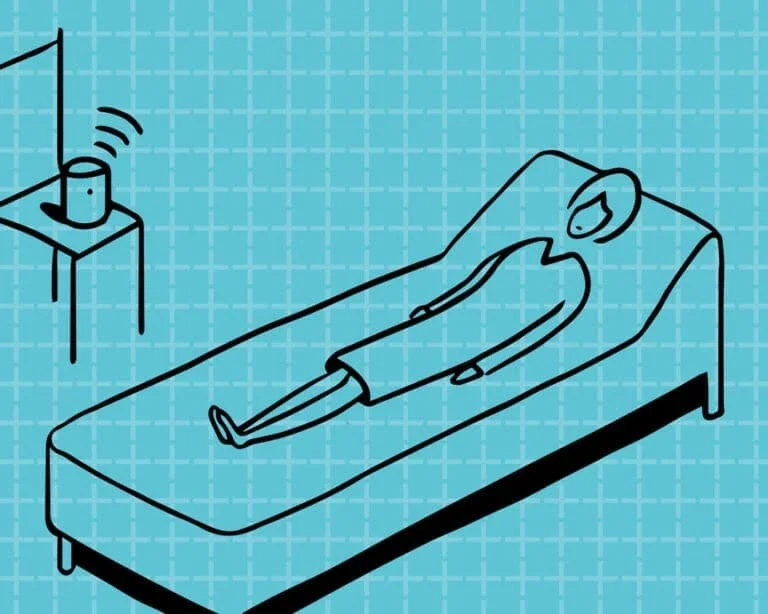
Solution Contours
Underwriters Laboratories, for example, a non-profit that conducts research and analyzes safety data was established after Thomas Edison laid wires that burst into flames. It developed the field of testing an observation which requires that human interaction be part of the engineered process, with public design elements. More recently, the auto industry’s requirements for crash testing reporting for example is an exemplar of data disclosure in the design, deployment, and launch phases, and for future monitoring and compliance, provided data needs are clear and the intellectual property of the firm is not compromised. Another reference point is participatory design in Scandinavia as the printing industry automated from the 1950s to 1970s and Open source models, frameworks, and tools to look over the shoulder of private entities. Governance and existing ethics and safety regulations and foundational thinking need to be embedded into decision flow, design structure and revisit methodologies.
Related Updates
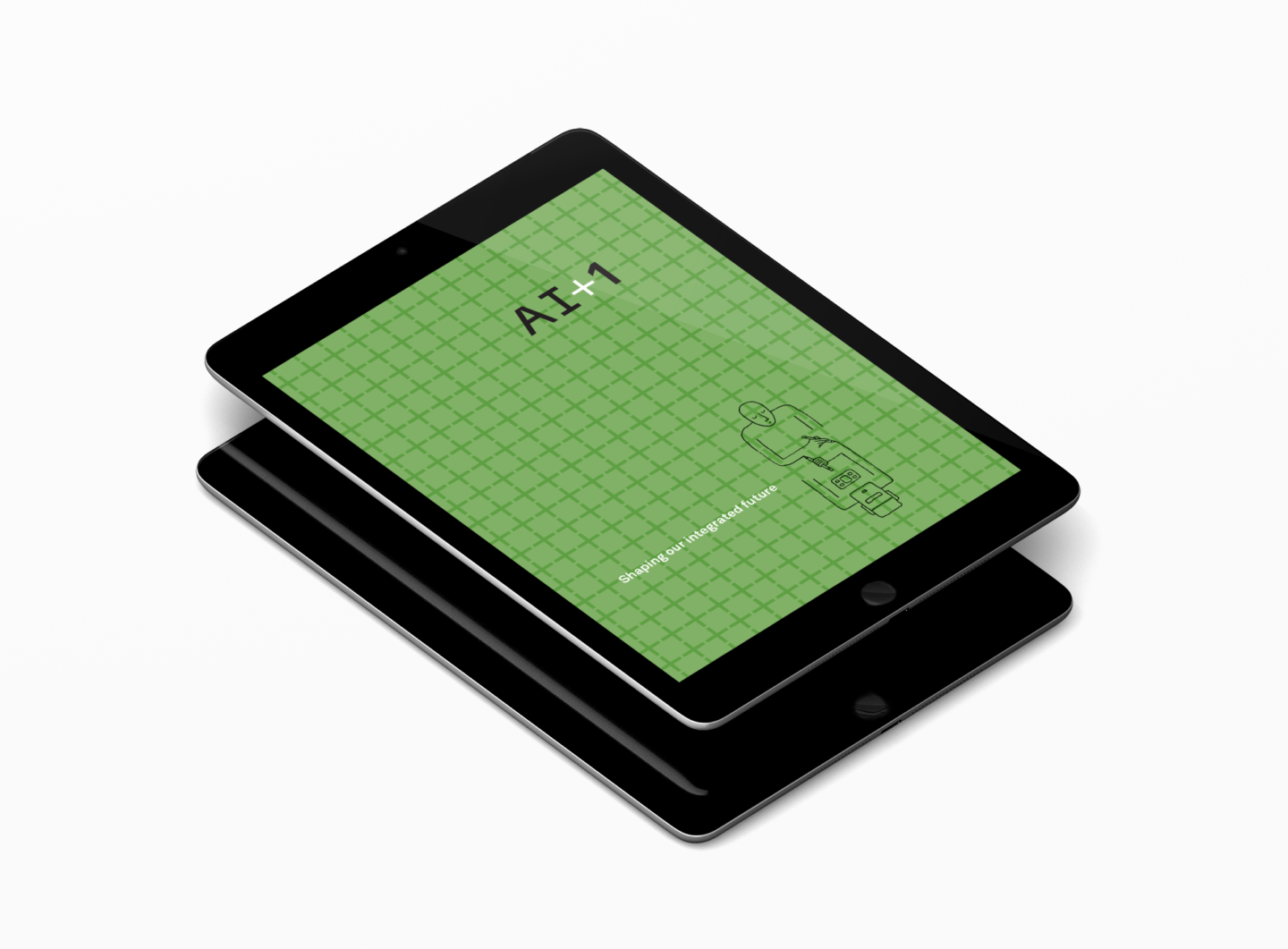
AI+1: Shaping Our Integrated Future
As we speak—and browse, and post photos, and move about—artificial intelligence is transforming the fabric of our lives. It is making life easier, better informed, healthier, more convenient. It also threatens to crimp our freedoms, worsen social disparities, and gives inordinate powers to unseen forces. Both AI’s virtues and risks have been on vivid display […]
More